Artificial intelligence seems to be doing it all! From driving cars to making mouth-watering pizzas, AI systems now augment decision-making processes in almost every industry. In some cases, they even call the shots. But amid all the developments, there is one roadblock that threatens to derail progress. And, no, we’re not talking about the creation of Norman, the world’s first psychopathic AI (although that is undoubtedly a questionable creation)! The bad apple that threatens to spoil the whole AI barrel is the inability of AI systems to explain themselves. A lot of the time, we don’t really know how or why an AI system produces the results that it does. Inexplicability is perhaps a good quality to have if you are a magician trying to astound your audience. However, in investment monitoring scenarios, where cold, hard facts take precedence over whimsy, it is something to be avoided. This is where explainable AI steps in to save the day. It adds much-needed clarity to the augmented process, thereby making it more trustworthy and reliable.
Conclusion - No more woo-woo credit monitoring
Explainable AI – The what, why, and how of it all

The What – AI’s black box explained
Inexplicability in artificial intelligence wasn’t always an issue. Earlier AI systems ran on rule-based algorithms and were, therefore, simpler and more transparent about their decision-making processes. Unfortunately, these standard
algorithms only have the capacity to process a limited number of data points. In investment-monitoring situations, this is rarely the case. The need for enhanced capability is what drove the rise of more advanced forms of AI such as neural networks and deep learning. These systems are modeled on the brain and can withstand a vast influx of data, even if it consists of millions of parameters. More importantly, they can find patterns and form connections within this data, making them adept at handling complicated scenarios.
However, just like the brain, these models are also nebulous because of their complex arrangement of ‘digital neurons’ and their interconnections. Compounding the enigma is the system’s ability to learn, grow, and direct itself like an actual living organism. Each neuron processes information and passes on its ‘learnings’ to the next. We on the outside, however, do not see the inner workings between these connections, what each neuron has learned, or the output between them. We are, therefore, left with the final output and a whole lot of guesswork as to how it got there.
What’s going on inside?
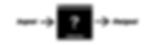
This is what AI pundits call the black box. The term exemplifies our inability to see what is going on inside these powerful systems. A classic example of this is Deep Patient, a diagnosing AI system designed by New York’s Mount Sinai hospital. Engineers trained Deep Patient using the hospital’s vast database of over 700,000 patient records. When tested against new records, the program proved adept at predicting a wide range of illnesses accurately. It even forecasted diseases that are notoriously difficult for physicians to predict such as schizophrenia. Since experts had no clue of the system’s methodology, they were left scratching their heads as to its possibility.
Explainable AI (or XAI) is simply the programming that exposes the rationale behind an augmented system’s final output. In essence, it removes the black box and replaces it with a transparent one, so we can see what is going on inside.
The Why – Importance of explainable AI
Checking the validity of decisions
So, does it really matter if we don’t know what’s going on inside the AI black box as long as we’re getting some answers? The problem here is not the ability of AI systems to produce an output but the validity of those outputs itself. Like human beings, AI networks can make mistakes. Unlike human beings, however, computer systems do not realize their mistakes, nor do they have the moral code needed to understand the implications of their decision-making.
What’s worse, unless we can see the reasoning behind a system’s output, there is no way of knowing if its logic is flawed or not. For example, let's suppose a neural network has been trained with a million images of cats and that most of these images have a copyright symbol on them. Now, when the system correctly identifies cat pictures, is it doing so based on a cat’s physical characteristics, or is it simply looking for the copyright symbol? If it is the latter, the system is producing the right results but for the wrong reasons. Down the road, its flawed logic is also bound to cause a few misidentifications. These nonsensical correlations can be avoided if we can see the equations and calculations behind the output.
Data bias
In addition to an inability to identify mistakes and flawed logic, AI systems are also dependent on the data they are fed. Recently, news articles claiming a correlation between psychopaths and black coffee drinkers went viral. Our human brains can laugh off this bit of data knowing that it paints an incomplete picture of a would-be psychopath. A computer system fed only with this information, however, can be tricked into thinking that all black coffee drinkers are psychopaths!
Admittedly, the stakes in the above scenario are pretty low. But what happens if the opposite is true? An example of AI automation going wrong with real-life ramifications is Amazon’s erstwhile AI recruiting tool. The company’s engineers aimed at building a system that would simplify the hiring process by automatically recommending candidates for a job. They trained the system using a decade worth of resumes previously received by the company.
The problem was that most of the resumes the company received for technical jobs were from male candidates (a reflection of the gender imbalance in the tech sector). Consequently, the system trained itself to downgrade resumes that had the word ‘female’ or ‘women’ in it. In essence, it did not recommend candidates in a gender-neutral way. Thankfully, the company scrapped the prejudiced recruiting program once they discovered its bias.
Legal regulations
Another development that underlines the need for XAI is the slow but steady rise in regulatory pressure. In 2016, the European Union released the GDPR (General Data Protection Rules) act. Article 22 of this act limits an organization’s ability to rely solely on decisions made by automated processes. Crucially, it also gives individuals affected by these automated judgments the right to question the decision-making process.
At present, there are no such regulations in place in the US. That’s not to say that some aren’t in the pipeline. In 2018, the New York City Council passed the Algorithmic Accountability Act. It mandates that all automated decisions be verifiable. Politicians are all set to re-introduce a bill with the same name and stipulations in the House and Senate this year. As AI systems become more and more omnipresent, it seems more a question of when, rather than if, more regulations controlling automated decisions happen.
The How – Stepping out of the black box
The simplest way to achieve AI transparency is to exclusively use ML programming that is inherently interpretable. Due to their linear nature, models using decision trees, logistic regression, and Bayesian classifiers have innate traceability to them. Industry experts fittingly call these systems ‘glass box models’. They are a great fit for data problems that are not very complex and therefore do not need bombastic algorithms to master them. Sometimes, simple works best. As a 14th-century philosopher put it, “The simplest solution is almost always the best solution”.
Of course, thornier data problems that have myriad parameters demand more sophisticated algorithms. These complex systems have a non-linear set up, making them more powerful but harder to interpret at the same time. And the more complex a system, the more inexplicable it is. The key then is to find a balanced approach that incorporates the advantages of both systems, ensuring smaller tradeoffs between accountability and ability.
One way to achieve this best-of-both-worlds objective is to use hybrid AI models which integrate the two systems. Here, programmers use advanced models for the predictive analysis part while standard algorithms perform an explanatory breakdown of it all. This creates a powerful, yet explainable system. A popular XAI technique that exemplifies this is LIME (Local Interpretable Model-Agnostic Explanations), which uses a linear ML model to interpret local datasets. Another upcoming example is the EBM (Explainable Boosting Machine) system that combines nascent ML techniques such as gradient boosting with interpretable ones such as linear regression.
Explainable AI in the investment monitoring industry
The investment sector faces a trifecta of challenges today – volatile markets that need constant supervision, a steady influx of data in need of analysis, and regulatory constraints that are progressively increasing. Given this, traditional manual methods of risk management just won’t cut it anymore. They are labor-intensive, time-consuming, and woefully inadequate. Augmented AI systems are, therefore, a game changer for this industry as they can cover all the bases quickly and efficiently.
On the flip side, the financial industry is also one of the most heavily regulated sectors worldwide. This means that the threat of regulatory fines hangs over financial institutions using black-box AI models like the sword of Damocles. This probably explains why only around 6% of investment managers currently use ML-based programming to augment their risk monitoring process even though over 75% of them acknowledge that it can play a bigger role in portfolio monitoring.
As a result, most of the AI in the investment monitoring industry is restricted to rule-based and scorecard-type programming. These models, though competent, cannot interconnect information and thus have less predictive capacity. Clearly, XAI has a great role to play in unlocking machine learning’s potential in investment monitoring. Fortunately, rapid developments in the field promise to make it the norm in investment circles.
How TRaiCE uses explainable AI
With TRaiCE, there is no pretense at omniscience or opaqueness. The platform’s powerful proprietary algorithms work hand-in-hand with XAI. It leverages internal, credit bureau, and other big data elements to not only trigger alerts but also to explain them. For example, if the application identifies a borrower as a high-risk customer, it also simultaneously displays the parameters that caused it to do so such as an external delinquency or tanking credit bureau scores. This way the logic is crystal clear and users don’t need to wonder about the veracity of the alert.
In addition, the platform does not use set-in-stone baseline rules. As a result, portfolio managers are not confined to using only traditional or historical credit monitoring parameters. Instead, they can now include alternate data metrics to build a more nuanced profile of their borrowers than was earlier possible. Having the freedom to configure their own guardrails also ensures that users can work collaboratively with the system to finetune its approach to investment monitoring. Crucially, it also allows them to uncover previously unknown credit-defaulting behaviors.
Over and above all this, the application trains itself continuously by observing user actions and juxtaposing them against portfolio performance. It then uses this data to train and augment its internal models to become more and more intuitive over time. It is this capacity to evolve, analyze, and network that makes the system adept at predicting future risk too. In this way, TRaiCE gives users a real-time and futuristic view of their portfolio’s performance using an amalgamation of sound, explainable logic and powerful programming.
Conclusion - No more woo-woo credit monitoring
Explainable AI in the investment industry clearly matters. It gives lenders the ability to improve their investment monitoring process without sacrificing any accountability. In other words, it avoids the woo-woo kind of credit monitoring that is based on mystique rather than fact. Just as ‘stepping out of the box’ personally or societally can help broaden your horizons, so it is with AI. Stepping out of the black box will no doubt expand AI’s reach and make it more ubiquitous in the investment industry.